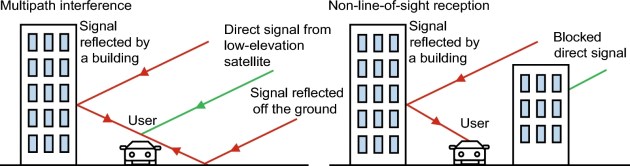
In an effort to tackle the persistent challenge posed by non-line-of-sight GNSS errors in urban jungle environments, researchers have introduced an innovative solution powered by AI.
By leveraging the Light Gradient Boosting Machine (LightGBM), the method analyses multiple GNSS signal features to accurately identify and differentiate NLOS errors.
The researchers say the breakthrough promises to significantly improve the precision and reliability of GNSS-based positioning systems, particularly for urban environments, where accuracy can be crucial.
In those urban environments, GNSS often struggle with signal obstructions caused by tall buildings, vehicles and other structures, leading to non-line-of-sight (NLOS) errors that cause positioning inaccuracies.
The need for real-time, effective solutions to detect and mitigate these NLOS errors has never been more urgent, as reliable GNSS-based positioning is vital for the development of smart cities and transportation networks.
The research, published in Satellite Navigation, introduces a machine learning approach to tackle the problem.
Researchers from Wuhan University, Southeast University, and Baidu developed a solution using the LightGBM, a powerful AI-driven model designed to detect and exclude NLOS-related inaccuracies.
The model’s performance was validated through dynamic real-world experiments conducted in Wuhan, China, proving its effectiveness in challenging urban environments.
The method involves the use of a fisheye camera to label GNSS signals as either line-of-sight (LOS) or NLOS, based on the visibility of satellites. The researchers then analysed a range of signal features, including signal-to-noise ratio, elevation angle, pseudo-range consistency, and phase consistency.
By identifying correlations between these features and signal types, the LightGBM model was able to accurately distinguish between LOS and NLOS signals, achieving an impressive 92% accuracy.
Compared to traditional methods such as XGBoost, the researchers say the approach delivered superior performance in both accuracy and computational efficiency.
The results show that excluding NLOS signals from GNSS solutions can lead to substantial improvements in positioning accuracy, especially in urban canyons where obstructions are common.
“This method represents a major leap forward in enhancing GNSS positioning in urban environments,” said lead researcher, Dr Xiaohong Zhang.
“By using machine learning to analyse multiple signal features, we’ve shown that excluding NLOS signals can significantly boost the accuracy and reliability of satellite-based navigation systems.”