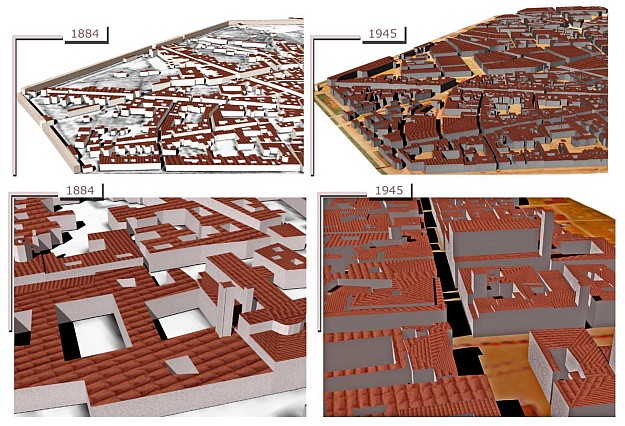
Inferring building height changes over time in Bologna using machine learning and maps from 1884 and 1945. Courtesy Farella E.M, et al./ MDPI Applied Sciences.
A methodology for deriving 4D building models using historical maps and machine learning has been proposed by a team of Russian and Italian scientists.
The researchers say that method should be useful “for understanding urban phenomena and changes contributing to defining our cities’ actual shapes”.
Their proposed technique uses geometric, neighbourhood and categorical attributes to predict building heights from analysis of 2D maps.
They say that when it comes to 3D/4D city modelling based on historical data, “the lack of building heights is a major obstacle for accurate space representation, analysis, visualization or simulations”.
So the team — from the Skolkovo Institute of Science and Technology (Skoltech) in Moscow and the 3DOM research unit of the Fondazione Bruno Kessler (FBK) in Trento, Italy — has used machine learning solutions to infer building heights from historical maps.
They tested the method on four maps of Trento (from the years 1851, 1887, 1908 and 1936) and Bologna (1884 and 1945).
The result was the assembly of multi-temporal (4D) versions of these cities.
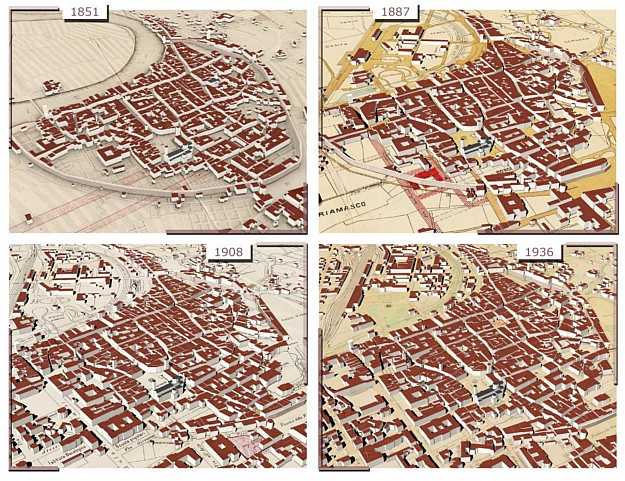
The changing landscape of Trento from 1851 to 1936. Courtesy Farella E.M, et al./ MDPI Applied Sciences.
“The implemented learning and predictive procedures tested on historical data have proven to be effective and promising for many other applications,” said Emre Ozdemir, a Skoltech and FBK Trento PhD student.
“Based on a few attributes for the prediction, it will soon be expanded to diverse real-life contexts with missing elevation data,” he added.
“The resulting models will be a great help in bridging the geospatial knowledge gap in past or remote situations.”
The research has been published in the MDPI Applied Sciences journal.
Stay up to date by getting stories like this delivered to your inbox.
Sign up to receive our free weekly Spatial Source newsletter.