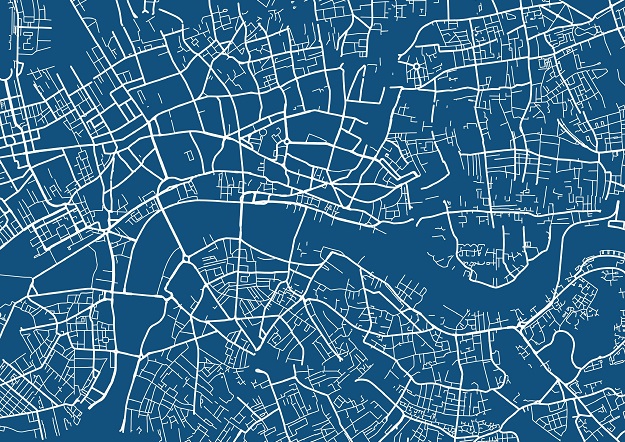
©stock.adobe.com/au/Mappingz
A system for mapping and visualising underground infrastructure assets such as pipes and cables, is being put to the test in the UK.
The concept, known as the Model for Underground Data Definition and Integration, or MUDDI, has been developed by data scientists in an Open Geospatial Consortium working group.
The aim of MUDDI is to visual subsurface assets and characterise the underground environments in which they sit, and define an encompassing data store. Once this is done, the data can be shared with those who need it and translated into easily understood maps.
Damage caused to underground assets from digging, and the need to alter plans when unexpected underground assets are found, costs nations hundreds of millions of dollars every year.
MUDDI was developed from extant data standards such as INSPIRE and CityGML. The aim is for it to eventually become a new international standard.
The UK Ordnance Survey’s Chris Popplestone said that MUDDI is an ideal example of a ‘harmonised data model’.
“The harmonised data model is that central target into which all of the source infrastructure data is transformed,” he said. “The source data comes in all different shapes and sizes, but it all gets transformed to this harmonised model.”
“We start off with a conceptual model of how this transformed data will look and we use various tools to focus that down into a physical model that can then be implemented as a data store. It’s a process from a high-level conceptual design down to an actual physical schema which can then be deployed by a development team.”
MUDDI has been trialled during pilot testing for the UK’s National Underground Asset Register (NUAR) program, which is led by the UK Government’s Geospatial Commission.
Two pilots were undertaken — one in London and the other in the northeast of England — and work has now begun on rolling out the system nationally under the lead of engineering consultancy, Atkins. That roll out of NUAR will lead to the development of a UK profile of MUDDI, which will be focused on the safe-digging use case.
Geospatial firm 1Spatial is providing the data transformation and data ingestion parts of the activity, with information collected from around 650 data or asset owners.
The task of shaping the MUDDI-based harmonised NUAR model is being undertaken by the Ordnance Survey, and involves building the data store and developing the software platform that will translate the data into a map-based interface. The idea is that field workers can highlight an area on the map, and it will instantly reveal the location of any relevant underground assets.
“The NUAR pilot was based on an earlier version of MUDDI, and the feedback we got from the pilot phase has fed into a new iteration of the MUDDI model, and that is now forming the basis of the national roll out of NUAR,” said Popplestone.
“There has been a lot of international involvement and that is being looked at very carefully for other international projects that are also planning to follow a similar approach.”
But the MUDDI concept will only be as good as the data fed into it, which is one of the biggest hurdles any such system faces.
“Because we know that is a risk, we have tried to make everything about the data model and the implementation of the data model as responsive as possible,” said Popplestone.
“Working under the Geospatial Commission we helped to build in flexibility to the UK’s ‘excavation profile,’ alongside strong governance structures, to try and streamline any additions that need to be made, so we don’t have to completely tear down data stores and rebuild them because we have found a new type of pipe.
“We have tried to make it quite modular and quite flexible.”
Popplestone says the subsurface asset data is a particular problem in the UK, where most of the country’s infrastructure is under the ownership of numerous private companies, each of which has its own (often disparate) data systems.
“That is part of what the data model is designed to solve,” he said. “There is potentially so much variation that we have to be able to make tweaks and add things that we haven’t come across in other data sets.”
“So, things like terminology can be quite different between different companies — we must make sure when we come across a new term it is a genuinely a new thing rather than something else that we have already modelled [but] called by a different name.”