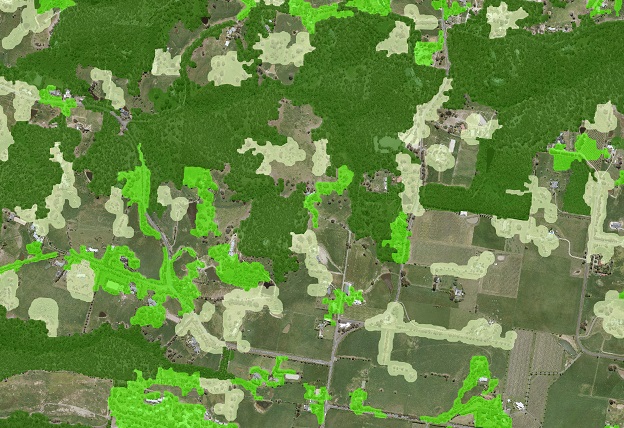
Vicmap Vegetation Tree Density shows the presence of woody vegetation and has been divided into three distinct density classes. The density classes show the spatial separation of woody vegetation of dense, medium and sparse. It has been derived from the Vicmap Vegetation Tree Extent dataset. All images © State of Victoria (DELWP).
Victoria’s DELWP has used machine learning techniques to refresh the state’s 20-year-old tree cover dataset.
By Cat Gilbert and John White (DELWP) and Caitlin Adams (FrontierSI)
Accurate and up-to-date vegetation mapping is critical for understanding urban heat in our cities, monitoring our environment and supporting emergency response and fire hazard assessment.
In Victoria, the Department of Environment, Land, Water and Planning (DELWP) recently completed a project to refresh its 20-year-old statewide tree cover dataset — Vicmap Vegetation Density.
Maintaining the vegetation density dataset has historically been a challenge because of the intensive manual-labour and costs involved. For the first time, DELWP has used emerging machine learning technology to update and create a Vicmap product, meaning more up-to-date and relevant data for customers.
The project applied machine learning technology to existing aerial imagery and elevation data to identify statewide tree canopy extent, statewide tree spatial density and individual tree points in urban areas.
To complete the project, DELWP’s Vicmap Spatial Services partnered with FrontierSI to update the vegetation datasets and used DELWP’s extensive collection of aerial imagery as the basis of the machine learning process. They also implemented an automated and repeatable process for refreshing the products regularly.
The Vicmap Tree Extent is now available at 20cm resolution, a 2,500-fold improvement in resolution compared to the previous 10m resolution and made possible only by combining high-resolution remote sensing data with emerging machine learning techniques. This improved resolution will enable users to conduct detailed, site-level analysis as well landscape-scale studies.
The datasets will also be available for 3D visualisation and analysis through the new Digital Twin Victoria Platform (https://www.land.vic.gov.au/maps-and-spatial/projects-and-programs/digital-twin-victoria) when it launches later this year.
Technique
The project leveraged two AI techniques belonging to the field of computer vision: semantic segmentation and object detection. In the digital image processing field, semantic segmentation enables the pixel-level classification in an image, meaning that it classifies the pixels into their corresponding classes. Object detection is a branch of machine learning focused on extracting meaningful patterns and objects from images. It classifies the patches of an image into different object classes and creates a bounding box around that object.
FrontierSI and a team from Orbica Ltd created training datasets that would enable the machine learning algorithm to learn how to identify the visual characteristics of tree canopies. Computer vision models were trained to classify pixels in DELWP’s 20cm imagery as trees/non-trees by showing the models many examples of how people identify areas of tree cover across Victoria. The trained model was then used to classify images across 43 of DELWP’s imagery projects, producing a statewide raster tree cover product.
The result was then generalised into a statewide density polygon vector layer, which categorised the spatial density of tree cover into Dense, Medium and Sparse categories.
The project also included identifying individual tree points in urban areas. Using the machine learning feature extraction (MLFE) proof of concept that DELWP conducted in 2018–19, an additional computer vision model was trained to identify individual trees in urban areas across metro Melbourne and four regional councils: Ballarat, Sale, Shepparton and Wangaratta. The tree points represent tree centres, along with their radial extent, and an estimated height from a digital surface model or canopy height model derived from associated LiDAR captures.
The statewide tree canopy extent was then evaluated by comparing it to human delineated extents or tree points and comparing the intersection over union values — a measurement of how well the model identified trees correctly. This was done for 12 regions across Victoria with different woody vegetation characteristics. The statewide product has an overall accuracy of 80%, but six of the test regions have accuracies above 95%, indicating that some regions performed better than others.
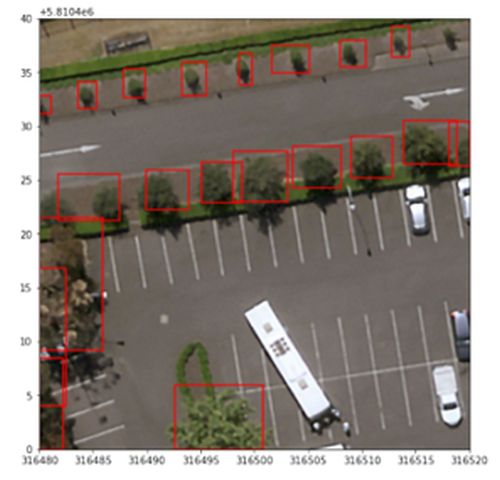
Human delineated tree extents helped to train and assess the accuracy of the Vicmap Vegetation Tree Urban machine learning model.
The project would not have been possible without the imagery archive held by DELWP’s Coordinated Imagery Program, which includes aerial imagery dating all the way back to the 1940s. The imagery archive is added to annually through proactive, coordinated data acquisition across Victorian program partners and represents the most comprehensive high-resolution imagery dataset for Victoria.
The team processed more than 35 terabytes of aerial imagery on Amazon Web Services’ cloud-computing services for the statewide products. Without this level of automation and scalability, the creation of the new products would have been impractical using traditional manual digitisation methods.
Lessons learned
The project encountered several challenges that produced useful insights.
Investment in the training datasets is key. The first lesson was that the quality of the training data is more important than the quantity.
Training data sets are critical inputs into the process. There are a small number of robust and open-source machine learning algorithms that can be successfully applied to similar sets of machine learning problems, but their success depends on the quality of the training datasets that they are given.
These training datasets also need to be localised to the attribute that they are being used to detect. For example, houses look different in many parts of India, America and Australia. A machine learning process to detect residential buildings that has been trained on one region and then applied to another will be full of errors.
The same applies to vegetation. In Victoria’s western Mallee, scrubland trees are short and sparse, adapted to the low rainfall conditions. But Victoria also hosts wet temperate rainforests. The model and the training datasets need to accommodate regional variation across the area of interest.
As the data is updated, continual assessment of the model’s performance is required. The accuracy of the machine learning model will need to be consistently reassessed to determine if it needs to be re-trained when new imagery is introduced to maintain the accuracy and performance of the model. This may mean that new imagery surveys include the parallel development of new validation data sets to be used for this purpose.
High resolution aerial photography must have a consistent level of quality across projects. The machine learning models implemented performed best where the imagery was consistent in quality. In some areas the photography varied in quality, resulting in a lower accuracy.
Human intervention is still required throughout the process. Understanding the level of post processing and human intervention and review throughout the process is critical. For example, categories were defined by updating the existing Vegetation product’s ruleset to suit the higher resolution product. This ensured that the new vegetation density dataset is like the existing Vicmap Vegetation product but has far greater detail. This process involved dozens of experts from across the field to provide input and discuss the pros and cons of different ways to define the ruleset.
Human input into the models gives them meaning and ensures they can be used and interpreted appropriately.

The machine learning model identified tree extents and assigned a confidence value to the outputs.
Future developments
The lessons from this project have already begun to inform how DELWP quality assures our aerial imagery, which will have affects back to imagery suppliers. Systematic processing errors in imagery which may have passed visual inspection — and be suitable for the use of imagery as a context layer — can become obvious and significant when the imagery is used in downstream ML-AI processes.
Internally, the Vicmap and Digital Twin Victoria programs in DELWP are investing in developing new data science capabilities and have created new dedicated data science roles. These staff are collaborating with peers across the Victorian public sector and with other jurisdictions through the Intergovernmental Committee on Surveying and Mapping (ICSM) to build up communities of practice for this rapidly evolving domain.
Finally, we know through the establishment in Victoria of the Geospatial Data and Analytics Panel, which has a new category dedicated to Geospatial Analytics, that the Australian market is progressing rapidly and offering a range of new and world-leading products and services.
We anticipate greater engagement between the public and private sector over the next few years as we work together to apply these emerging spatial technologies to the most pressing and complex problems in our communities.
Cat Gilbert and John White are with Vicmap Spatial Services, Department of Environment, Land, Water and Planning, and Caitlin Adams is with FrontierSI.
The datasets are available on datashare.maps.vic.gov.au. To gain access to imagery and elevation datasets, contact coordinated.imagery@delwp.vic.gov.au. If you want to learn more, visit the VicMap team at the Locate Conference 2022.
This article was first published in the Feb/Mar 2022 issue of Position magazine.
Stay up to date by getting stories like this delivered to your inbox.
Sign up to receive our free weekly Spatial Source newsletter.